Ming Chen
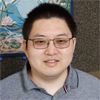
- Assistant Professor | Physical/Theoretical Chemistry
- Email: chen4116@purdue.edu
- Phone: 765 49-63737
- Office: 221A WTHR
- Professor Ming Chen's individual homepage
Theoretical chemistry meets data sciences. Our research group is interested in lifting obstacles in theoretical and computational chemistry with machine learning techniques. Our research focuses on understanding biomolecule conformational dynamics and modeling electronic structures of complex materials.
We use molecular dynamics to study biomolecule conformational dynamics like protein folding, drug binding, and protein-protein interactions. However, the timescale and length scale of molecular dynamics simulations are limited. Machine learning enlightens an alternative pathway to overcome the limitations of molecular dynamics by developing machine learning based enhanced sampling methods and machine learning based coarse-grained models.
Ab initio electronic structure methods are important approaches for studying various properties of materials. However, these methods often suffer from high computational costs, especially high scaling. We are developing a family of stochastic electronic structure methods which can significantly reduce the scaling (and the costs) of ab initio electronic structure calculations by introducing stochastic sampling to electronic structure calculations. Although stochastic electronic structure methods are efficient, noises are introduced in all calculated properties and these methods can only calculate certain physical properties efficiently. We are developing machine learning based methods to learn physical quantities from noisy calculation results and to recover other physical quantities that can not be evaluated efficiently with stochastic electronic structure methods.
Education
- B.S., Peking University, 2008
- Ph.D., New York University, 2016
- Postdoctoral Fellow, University of California, Berkeley, 2018
- Postdoctoral Fellow, Lawrence Berkeley National Laboratory, 2021